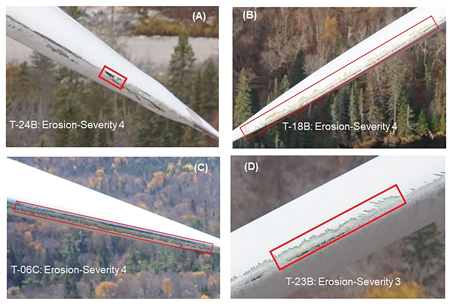
The ability to detect and quantify leading edge erosion (LEE) on wind turbine blades is important to improve power efficiency and to develop predictive maintenance strategies that can be used to identify damage early, allowing more efficient, proactive repairs. LEE leads to an increase in roughness, an increase in airfoil drag, local variations in the boundary layer, and, above all, changes in the aerodynamic performance of the blade, which in turn affects the noise produced by the blade as the turbine operates.
By Obdulia Ley, Subject Matter Expert in Acoustic Emission, Mistras Group, USA