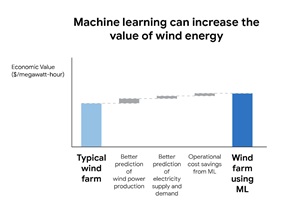
Based on these predictions, the model recommends how to make optimal hourly delivery commitments to the power grid a full day in advance. This is important, because energy sources that can be scheduled (i.e. can deliver a set amount of electricity at a set time) are often more valuable to the grid. Their use of machine learning across their wind farms has produced positive results. To date, machine learning has boosted the value of their wind energy by roughly 20%, compared to the baseline scenario of no time-based commitments to the grid.